#algorithmic bias
Explore tagged Tumblr posts
Text
The surprising truth about data-driven dictatorships
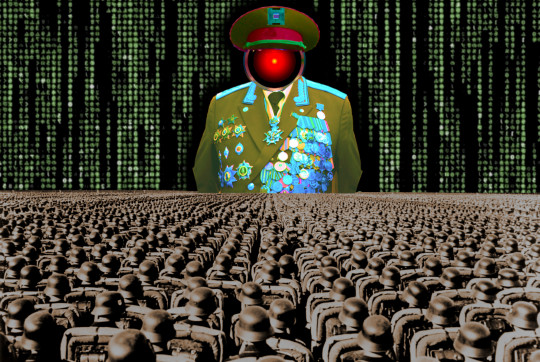
Here’s the “dictator’s dilemma”: they want to block their country’s frustrated elites from mobilizing against them, so they censor public communications; but they also want to know what their people truly believe, so they can head off simmering resentments before they boil over into regime-toppling revolutions.
These two strategies are in tension: the more you censor, the less you know about the true feelings of your citizens and the easier it will be to miss serious problems until they spill over into the streets (think: the fall of the Berlin Wall or Tunisia before the Arab Spring). Dictators try to square this circle with things like private opinion polling or petition systems, but these capture a small slice of the potentially destabiziling moods circulating in the body politic.
Enter AI: back in 2018, Yuval Harari proposed that AI would supercharge dictatorships by mining and summarizing the public mood — as captured on social media — allowing dictators to tack into serious discontent and diffuse it before it erupted into unequenchable wildfire:
https://www.theatlantic.com/magazine/archive/2018/10/yuval-noah-harari-technology-tyranny/568330/
Harari wrote that “the desire to concentrate all information and power in one place may become [dictators] decisive advantage in the 21st century.” But other political scientists sharply disagreed. Last year, Henry Farrell, Jeremy Wallace and Abraham Newman published a thoroughgoing rebuttal to Harari in Foreign Affairs:
https://www.foreignaffairs.com/world/spirals-delusion-artificial-intelligence-decision-making
They argued that — like everyone who gets excited about AI, only to have their hopes dashed — dictators seeking to use AI to understand the public mood would run into serious training data bias problems. After all, people living under dictatorships know that spouting off about their discontent and desire for change is a risky business, so they will self-censor on social media. That’s true even if a person isn’t afraid of retaliation: if you know that using certain words or phrases in a post will get it autoblocked by a censorbot, what’s the point of trying to use those words?
The phrase “Garbage In, Garbage Out” dates back to 1957. That’s how long we’ve known that a computer that operates on bad data will barf up bad conclusions. But this is a very inconvenient truth for AI weirdos: having given up on manually assembling training data based on careful human judgment with multiple review steps, the AI industry “pivoted” to mass ingestion of scraped data from the whole internet.
But adding more unreliable data to an unreliable dataset doesn’t improve its reliability. GIGO is the iron law of computing, and you can’t repeal it by shoveling more garbage into the top of the training funnel:
https://memex.craphound.com/2018/05/29/garbage-in-garbage-out-machine-learning-has-not-repealed-the-iron-law-of-computer-science/
When it comes to “AI” that’s used for decision support — that is, when an algorithm tells humans what to do and they do it — then you get something worse than Garbage In, Garbage Out — you get Garbage In, Garbage Out, Garbage Back In Again. That’s when the AI spits out something wrong, and then another AI sucks up that wrong conclusion and uses it to generate more conclusions.
To see this in action, consider the deeply flawed predictive policing systems that cities around the world rely on. These systems suck up crime data from the cops, then predict where crime is going to be, and send cops to those “hotspots” to do things like throw Black kids up against a wall and make them turn out their pockets, or pull over drivers and search their cars after pretending to have smelled cannabis.
The problem here is that “crime the police detected” isn’t the same as “crime.” You only find crime where you look for it. For example, there are far more incidents of domestic abuse reported in apartment buildings than in fully detached homes. That’s not because apartment dwellers are more likely to be wife-beaters: it’s because domestic abuse is most often reported by a neighbor who hears it through the walls.
So if your cops practice racially biased policing (I know, this is hard to imagine, but stay with me /s), then the crime they detect will already be a function of bias. If you only ever throw Black kids up against a wall and turn out their pockets, then every knife and dime-bag you find in someone’s pockets will come from some Black kid the cops decided to harass.
That’s life without AI. But now let’s throw in predictive policing: feed your “knives found in pockets” data to an algorithm and ask it to predict where there are more knives in pockets, and it will send you back to that Black neighborhood and tell you do throw even more Black kids up against a wall and search their pockets. The more you do this, the more knives you’ll find, and the more you’ll go back and do it again.
This is what Patrick Ball from the Human Rights Data Analysis Group calls “empiricism washing”: take a biased procedure and feed it to an algorithm, and then you get to go and do more biased procedures, and whenever anyone accuses you of bias, you can insist that you’re just following an empirical conclusion of a neutral algorithm, because “math can’t be racist.”
HRDAG has done excellent work on this, finding a natural experiment that makes the problem of GIGOGBI crystal clear. The National Survey On Drug Use and Health produces the gold standard snapshot of drug use in America. Kristian Lum and William Isaac took Oakland’s drug arrest data from 2010 and asked Predpol, a leading predictive policing product, to predict where Oakland’s 2011 drug use would take place.
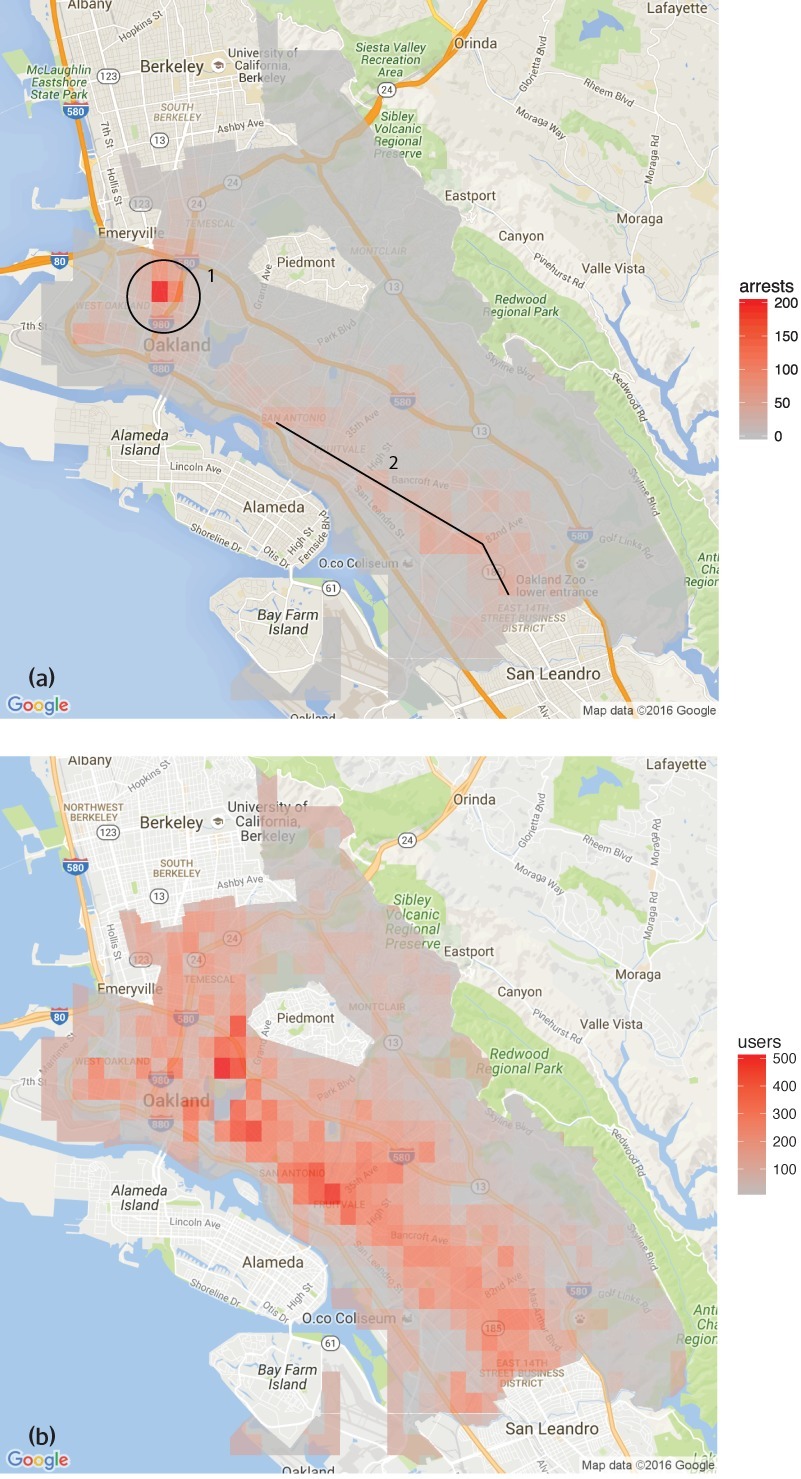
[Image ID: (a) Number of drug arrests made by Oakland police department, 2010. (1) West Oakland, (2) International Boulevard. (b) Estimated number of drug users, based on 2011 National Survey on Drug Use and Health]
Then, they compared those predictions to the outcomes of the 2011 survey, which shows where actual drug use took place. The two maps couldn’t be more different:
https://rss.onlinelibrary.wiley.com/doi/full/10.1111/j.1740-9713.2016.00960.x
Predpol told cops to go and look for drug use in a predominantly Black, working class neighborhood. Meanwhile the NSDUH survey showed the actual drug use took place all over Oakland, with a higher concentration in the Berkeley-neighboring student neighborhood.
What’s even more vivid is what happens when you simulate running Predpol on the new arrest data that would be generated by cops following its recommendations. If the cops went to that Black neighborhood and found more drugs there and told Predpol about it, the recommendation gets stronger and more confident.
In other words, GIGOGBI is a system for concentrating bias. Even trace amounts of bias in the original training data get refined and magnified when they are output though a decision support system that directs humans to go an act on that output. Algorithms are to bias what centrifuges are to radioactive ore: a way to turn minute amounts of bias into pluripotent, indestructible toxic waste.
There’s a great name for an AI that’s trained on an AI’s output, courtesy of Jathan Sadowski: “Habsburg AI.”
And that brings me back to the Dictator’s Dilemma. If your citizens are self-censoring in order to avoid retaliation or algorithmic shadowbanning, then the AI you train on their posts in order to find out what they’re really thinking will steer you in the opposite direction, so you make bad policies that make people angrier and destabilize things more.
Or at least, that was Farrell(et al)’s theory. And for many years, that’s where the debate over AI and dictatorship has stalled: theory vs theory. But now, there’s some empirical data on this, thanks to the “The Digital Dictator’s Dilemma,” a new paper from UCSD PhD candidate Eddie Yang:
https://www.eddieyang.net/research/DDD.pdf
Yang figured out a way to test these dueling hypotheses. He got 10 million Chinese social media posts from the start of the pandemic, before companies like Weibo were required to censor certain pandemic-related posts as politically sensitive. Yang treats these posts as a robust snapshot of public opinion: because there was no censorship of pandemic-related chatter, Chinese users were free to post anything they wanted without having to self-censor for fear of retaliation or deletion.
Next, Yang acquired the censorship model used by a real Chinese social media company to decide which posts should be blocked. Using this, he was able to determine which of the posts in the original set would be censored today in China.
That means that Yang knows that the “real” sentiment in the Chinese social media snapshot is, and what Chinese authorities would believe it to be if Chinese users were self-censoring all the posts that would be flagged by censorware today.
From here, Yang was able to play with the knobs, and determine how “preference-falsification” (when users lie about their feelings) and self-censorship would give a dictatorship a misleading view of public sentiment. What he finds is that the more repressive a regime is — the more people are incentivized to falsify or censor their views — the worse the system gets at uncovering the true public mood.
What’s more, adding additional (bad) data to the system doesn’t fix this “missing data” problem. GIGO remains an iron law of computing in this context, too.
But it gets better (or worse, I guess): Yang models a “crisis” scenario in which users stop self-censoring and start articulating their true views (because they’ve run out of fucks to give). This is the most dangerous moment for a dictator, and depending on the dictatorship handles it, they either get another decade or rule, or they wake up with guillotines on their lawns.
But “crisis” is where AI performs the worst. Trained on the “status quo” data where users are continuously self-censoring and preference-falsifying, AI has no clue how to handle the unvarnished truth. Both its recommendations about what to censor and its summaries of public sentiment are the least accurate when crisis erupts.
But here’s an interesting wrinkle: Yang scraped a bunch of Chinese users’ posts from Twitter — which the Chinese government doesn’t get to censor (yet) or spy on (yet) — and fed them to the model. He hypothesized that when Chinese users post to American social media, they don’t self-censor or preference-falsify, so this data should help the model improve its accuracy.
He was right — the model got significantly better once it ingested data from Twitter than when it was working solely from Weibo posts. And Yang notes that dictatorships all over the world are widely understood to be scraping western/northern social media.
But even though Twitter data improved the model’s accuracy, it was still wildly inaccurate, compared to the same model trained on a full set of un-self-censored, un-falsified data. GIGO is not an option, it’s the law (of computing).
Writing about the study on Crooked Timber, Farrell notes that as the world fills up with “garbage and noise” (he invokes Philip K Dick’s delighted coinage “gubbish”), “approximately correct knowledge becomes the scarce and valuable resource.”
https://crookedtimber.org/2023/07/25/51610/
This “probably approximately correct knowledge” comes from humans, not LLMs or AI, and so “the social applications of machine learning in non-authoritarian societies are just as parasitic on these forms of human knowledge production as authoritarian governments.”
The Clarion Science Fiction and Fantasy Writers’ Workshop summer fundraiser is almost over! I am an alum, instructor and volunteer board member for this nonprofit workshop whose alums include Octavia Butler, Kim Stanley Robinson, Bruce Sterling, Nalo Hopkinson, Kameron Hurley, Nnedi Okorafor, Lucius Shepard, and Ted Chiang! Your donations will help us subsidize tuition for students, making Clarion — and sf/f — more accessible for all kinds of writers.
Libro.fm is the indie-bookstore-friendly, DRM-free audiobook alternative to Audible, the Amazon-owned monopolist that locks every book you buy to Amazon forever. When you buy a book on Libro, they share some of the purchase price with a local indie bookstore of your choosing (Libro is the best partner I have in selling my own DRM-free audiobooks!). As of today, Libro is even better, because it’s available in five new territories and currencies: Canada, the UK, the EU, Australia and New Zealand!
[Image ID: An altered image of the Nuremberg rally, with ranked lines of soldiers facing a towering figure in a many-ribboned soldier's coat. He wears a high-peaked cap with a microchip in place of insignia. His head has been replaced with the menacing red eye of HAL9000 from Stanley Kubrick's '2001: A Space Odyssey.' The sky behind him is filled with a 'code waterfall' from 'The Matrix.']
Image: Cryteria (modified) https://commons.wikimedia.org/wiki/File:HAL9000.svg
CC BY 3.0 https://creativecommons.org/licenses/by/3.0/deed.en
—
Raimond Spekking (modified) https://commons.wikimedia.org/wiki/File:Acer_Extensa_5220_-_Columbia_MB_06236-1N_-_Intel_Celeron_M_530_-_SLA2G_-_in_Socket_479-5029.jpg
CC BY-SA 4.0 https://creativecommons.org/licenses/by-sa/4.0/deed.en
—
Russian Airborne Troops (modified) https://commons.wikimedia.org/wiki/File:Vladislav_Achalov_at_the_Airborne_Troops_Day_in_Moscow_%E2%80%93_August_2,_2008.jpg
“Soldiers of Russia” Cultural Center (modified) https://commons.wikimedia.org/wiki/File:Col._Leonid_Khabarov_in_an_everyday_service_uniform.JPG
CC BY-SA 3.0 https://creativecommons.org/licenses/by-sa/3.0/deed.en
#pluralistic#habsburg ai#self censorship#henry farrell#digital dictatorships#machine learning#dictator's dilemma#eddie yang#preference falsification#political science#training bias#scholarship#spirals of delusion#algorithmic bias#ml#Fully automated data driven authoritarianism#authoritarianism#gigo#garbage in garbage out garbage back in#gigogbi#yuval noah harari#gubbish#pkd#philip k dick#phildickian
831 notes
·
View notes
Text

there's no salvation in the algorithm
#art#retrofuture#design#collage#aesthetic#cyberpunk#black and white#glitch#text#typeface#algorithmic bias#free palestine
137 notes
·
View notes
Text
For my own mental health, I have a rule of thumb about communities, whether a political party or a discord group:
Never join a community that is only against something, stick to communities that are for something.
If the only thing you have in common with the people around you is a shared Hate, that's all you're going to get. It rots your soul from the inside to make yourself addicted to recreational Anger as your main form of socialization and entertainment. Social media algorithms tend to amplify those groups though, because people keeping themselves perpetually pissed-off about their issue of choice keep coming back for more, and the algorithm is designed to increase "engagement" by any means necessary.
Like, join a group for your hobby, or your favorite band, or an activist cause you believe in, or at least just go have fun somewhere. Go find people who make you feel good to be around.
Stay away from groups that start making up fake stories to make themselves mad when they run out of real material...
#social media#hate groups#algorithmic bias#the “JustNoMIL” subreddit was like this#sure everybody has an evil mother in law story#but they ran out of those years ago#and its basically a creative-writing forum#with a “make me mad at this stranger's family” theme
16 notes
·
View notes
Text
20 notes
·
View notes
Note
Do you know what happened to @gay-bucky-barnes blog? It’s not showing up for me
i think it was deleted by tumblr's algorithm again. betting bc of coded biases against queer content. givim a follow again @gaybuckybarnesagain pls.
5 notes
·
View notes
Text
I honestly $#@&ing hate it how Youtube's algorithm effectively prevents you from finding anything new, anything outside the things you usually watch. It traps you in a bubble you can't get out of, and the requirement to turn on the viewing history if you want to have any videos at all on the home page made the effect much stronger than it was before. It's so boring to watch it these days because you simply can't come across something interesting you didn't know about
#also why aren't people even talking about this??#this is a huge issue#youtube#social media#algorithm#algorithmic bias#echo chamber
2 notes
·
View notes
Text
AI Revolution: Balancing Benefits and Dangers
Not too long ago, I was conversing with one of our readers about artificial intelligence. They found it humorous that I believe we are more productive using ChatGPT and other generic AI solutions. Another reader expressed confidence that AI would not take over the music industry because it could never replace live performances. I also spoke with someone who embraced a deep fear of all things AI,…
#AI accountability#AI in healthcare#AI regulation#AI risks#AI transparency#algorithmic bias#artificial intelligence#automation#data privacy#ethical AI#generative AI#job displacement#machine learning#predictive policing#social implications of AI
0 notes
Text
Hypothetical AI election disinformation risks vs real AI harms
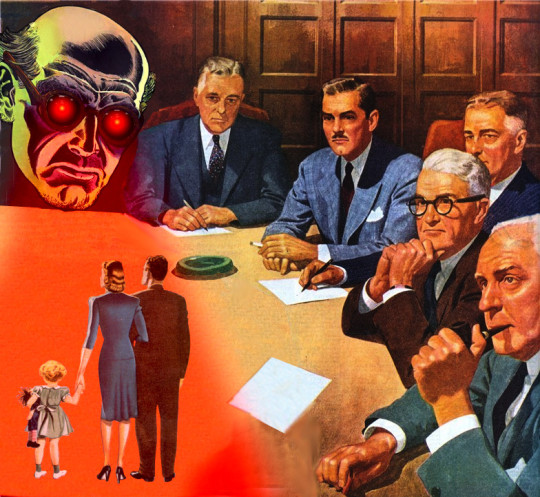
I'm on tour with my new novel The Bezzle! Catch me TONIGHT (Feb 27) in Portland at Powell's. Then, onto Phoenix (Changing Hands, Feb 29), Tucson (Mar 9-12), and more!
You can barely turn around these days without encountering a think-piece warning of the impending risk of AI disinformation in the coming elections. But a recent episode of This Machine Kills podcast reminds us that these are hypothetical risks, and there is no shortage of real AI harms:
https://soundcloud.com/thismachinekillspod/311-selling-pickaxes-for-the-ai-gold-rush
The algorithmic decision-making systems that increasingly run the back-ends to our lives are really, truly very bad at doing their jobs, and worse, these systems constitute a form of "empiricism-washing": if the computer says it's true, it must be true. There's no such thing as racist math, you SJW snowflake!
https://slate.com/news-and-politics/2019/02/aoc-algorithms-racist-bias.html
Nearly 1,000 British postmasters were wrongly convicted of fraud by Horizon, the faulty AI fraud-hunting system that Fujitsu provided to the Royal Mail. They had their lives ruined by this faulty AI, many went to prison, and at least four of the AI's victims killed themselves:
https://en.wikipedia.org/wiki/British_Post_Office_scandal
Tenants across America have seen their rents skyrocket thanks to Realpage's landlord price-fixing algorithm, which deployed the time-honored defense: "It's not a crime if we commit it with an app":
https://www.propublica.org/article/doj-backs-tenants-price-fixing-case-big-landlords-real-estate-tech
Housing, you'll recall, is pretty foundational in the human hierarchy of needs. Losing your home – or being forced to choose between paying rent or buying groceries or gas for your car or clothes for your kid – is a non-hypothetical, widespread, urgent problem that can be traced straight to AI.
Then there's predictive policing: cities across America and the world have bought systems that purport to tell the cops where to look for crime. Of course, these systems are trained on policing data from forces that are seeking to correct racial bias in their practices by using an algorithm to create "fairness." You feed this algorithm a data-set of where the police had detected crime in previous years, and it predicts where you'll find crime in the years to come.
But you only find crime where you look for it. If the cops only ever stop-and-frisk Black and brown kids, or pull over Black and brown drivers, then every knife, baggie or gun they find in someone's trunk or pockets will be found in a Black or brown person's trunk or pocket. A predictive policing algorithm will naively ingest this data and confidently assert that future crimes can be foiled by looking for more Black and brown people and searching them and pulling them over.
Obviously, this is bad for Black and brown people in low-income neighborhoods, whose baseline risk of an encounter with a cop turning violent or even lethal. But it's also bad for affluent people in affluent neighborhoods – because they are underpoliced as a result of these algorithmic biases. For example, domestic abuse that occurs in full detached single-family homes is systematically underrepresented in crime data, because the majority of domestic abuse calls originate with neighbors who can hear the abuse take place through a shared wall.
But the majority of algorithmic harms are inflicted on poor, racialized and/or working class people. Even if you escape a predictive policing algorithm, a facial recognition algorithm may wrongly accuse you of a crime, and even if you were far away from the site of the crime, the cops will still arrest you, because computers don't lie:
https://www.cbsnews.com/sacramento/news/texas-macys-sunglass-hut-facial-recognition-software-wrongful-arrest-sacramento-alibi/
Trying to get a low-waged service job? Be prepared for endless, nonsensical AI "personality tests" that make Scientology look like NASA:
https://futurism.com/mandatory-ai-hiring-tests
Service workers' schedules are at the mercy of shift-allocation algorithms that assign them hours that ensure that they fall just short of qualifying for health and other benefits. These algorithms push workers into "clopening" – where you close the store after midnight and then open it again the next morning before 5AM. And if you try to unionize, another algorithm – that spies on you and your fellow workers' social media activity – targets you for reprisals and your store for closure.
If you're driving an Amazon delivery van, algorithm watches your eyeballs and tells your boss that you're a bad driver if it doesn't like what it sees. If you're working in an Amazon warehouse, an algorithm decides if you've taken too many pee-breaks and automatically dings you:
https://pluralistic.net/2022/04/17/revenge-of-the-chickenized-reverse-centaurs/
If this disgusts you and you're hoping to use your ballot to elect lawmakers who will take up your cause, an algorithm stands in your way again. "AI" tools for purging voter rolls are especially harmful to racialized people – for example, they assume that two "Juan Gomez"es with a shared birthday in two different states must be the same person and remove one or both from the voter rolls:
https://www.cbsnews.com/news/eligible-voters-swept-up-conservative-activists-purge-voter-rolls/
Hoping to get a solid education, the sort that will keep you out of AI-supervised, precarious, low-waged work? Sorry, kiddo: the ed-tech system is riddled with algorithms. There's the grifty "remote invigilation" industry that watches you take tests via webcam and accuses you of cheating if your facial expressions fail its high-tech phrenology standards:
https://pluralistic.net/2022/02/16/unauthorized-paper/#cheating-anticheat
All of these are non-hypothetical, real risks from AI. The AI industry has proven itself incredibly adept at deflecting interest from real harms to hypothetical ones, like the "risk" that the spicy autocomplete will become conscious and take over the world in order to convert us all to paperclips:
https://pluralistic.net/2023/11/27/10-types-of-people/#taking-up-a-lot-of-space
Whenever you hear AI bosses talking about how seriously they're taking a hypothetical risk, that's the moment when you should check in on whether they're doing anything about all these longstanding, real risks. And even as AI bosses promise to fight hypothetical election disinformation, they continue to downplay or ignore the non-hypothetical, here-and-now harms of AI.
There's something unseemly – and even perverse – about worrying so much about AI and election disinformation. It plays into the narrative that kicked off in earnest in 2016, that the reason the electorate votes for manifestly unqualified candidates who run on a platform of bald-faced lies is that they are gullible and easily led astray.
But there's another explanation: the reason people accept conspiratorial accounts of how our institutions are run is because the institutions that are supposed to be defending us are corrupt and captured by actual conspiracies:
https://memex.craphound.com/2019/09/21/republic-of-lies-the-rise-of-conspiratorial-thinking-and-the-actual-conspiracies-that-fuel-it/
The party line on conspiratorial accounts is that these institutions are good, actually. Think of the rebuttal offered to anti-vaxxers who claimed that pharma giants were run by murderous sociopath billionaires who were in league with their regulators to kill us for a buck: "no, I think you'll find pharma companies are great and superbly regulated":
https://pluralistic.net/2023/09/05/not-that-naomi/#if-the-naomi-be-klein-youre-doing-just-fine
Institutions are profoundly important to a high-tech society. No one is capable of assessing all the life-or-death choices we make every day, from whether to trust the firmware in your car's anti-lock brakes, the alloys used in the structural members of your home, or the food-safety standards for the meal you're about to eat. We must rely on well-regulated experts to make these calls for us, and when the institutions fail us, we are thrown into a state of epistemological chaos. We must make decisions about whether to trust these technological systems, but we can't make informed choices because the one thing we're sure of is that our institutions aren't trustworthy.
Ironically, the long list of AI harms that we live with every day are the most important contributor to disinformation campaigns. It's these harms that provide the evidence for belief in conspiratorial accounts of the world, because each one is proof that the system can't be trusted. The election disinformation discourse focuses on the lies told – and not why those lies are credible.
That's because the subtext of election disinformation concerns is usually that the electorate is credulous, fools waiting to be suckered in. By refusing to contemplate the institutional failures that sit upstream of conspiracism, we can smugly locate the blame with the peddlers of lies and assume the mantle of paternalistic protectors of the easily gulled electorate.
But the group of people who are demonstrably being tricked by AI is the people who buy the horrifically flawed AI-based algorithmic systems and put them into use despite their manifest failures.
As I've written many times, "we're nowhere near a place where bots can steal your job, but we're certainly at the point where your boss can be suckered into firing you and replacing you with a bot that fails at doing your job"
https://pluralistic.net/2024/01/15/passive-income-brainworms/#four-hour-work-week
The most visible victims of AI disinformation are the people who are putting AI in charge of the life-chances of millions of the rest of us. Tackle that AI disinformation and its harms, and we'll make conspiratorial claims about our institutions being corrupt far less credible.
If you'd like an essay-formatted version of this post to read or share, here's a link to it on pluralistic.net, my surveillance-free, ad-free, tracker-free blog:
https://pluralistic.net/2024/02/27/ai-conspiracies/#epistemological-collapse
Image: Cryteria (modified) https://commons.wikimedia.org/wiki/File:HAL9000.svg
CC BY 3.0 https://creativecommons.org/licenses/by/3.0/deed.en
#pluralistic#ai#disinformation#algorithmic bias#elections#election disinformation#conspiratorialism#paternalism#this machine kills#Horizon#the rents too damned high#weaponized shelter#predictive policing#fr#facial recognition#labor#union busting#union avoidance#standardized testing#hiring#employment#remote invigilation
146 notes
·
View notes
Text
Navigating the complexities of AI and automation demands more than just technical prowess; it requires ethical leadership.
My latest blog post delves into building an "ethical algorithm" for your organization, addressing bias, the future of work, and maintaining human-centered values in a rapidly evolving technological landscape. Leaders at all levels will find practical strategies and thought-provoking insights.
Read more and subscribe for ongoing leadership guidance.
#Future Of Work#Ethical AI Leadership#Responsible Technology Adoption#Human Centered Values#Algorithmic Bias#Jerry Justice#TAImotivations
0 notes
Text
@staff the chronological timeline is why I'm here. It's a curated experience, not one where I am suddenly forced to see content I did not consent to, everything in my timeline is stuff and people I like. There already is an algo timeline, by all means tweak that, but if you take away the chrono timeline I will be gone. I will miss you all 😥
Tumblr’s Core Product Strategy
Here at Tumblr, we’ve been working hard on reorganizing how we work in a bid to gain more users. A larger user base means a more sustainable company, and means we get to stick around and do this thing with you all a bit longer. What follows is the strategy we're using to accomplish the goal of user growth. The @labs group has published a bit already, but this is bigger. We’re publishing it publicly for the first time, in an effort to work more transparently with all of you in the Tumblr community. This strategy provides guidance amid limited resources, allowing our teams to focus on specific key areas to ensure Tumblr’s future.
The Diagnosis
In order for Tumblr to grow, we need to fix the core experience that makes Tumblr a useful place for users. The underlying problem is that Tumblr is not easy to use. Historically, we have expected users to curate their feeds and lean into curating their experience. But this expectation introduces friction to the user experience and only serves a small portion of our audience.
Tumblr’s competitive advantage lies in its unique content and vibrant communities. As the forerunner of internet culture, Tumblr encompasses a wide range of interests, such as entertainment, art, gaming, fandom, fashion, and music. People come to Tumblr to immerse themselves in this culture, making it essential for us to ensure a seamless connection between people and content.
To guarantee Tumblr’s continued success, we’ve got to prioritize fostering that seamless connection between people and content. This involves attracting and retaining new users and creators, nurturing their growth, and encouraging frequent engagement with the platform.
Our Guiding Principles
To enhance Tumblr’s usability, we must address these core guiding principles.
Expand the ways new users can discover and sign up for Tumblr.
Provide high-quality content with every app launch.
Facilitate easier user participation in conversations.
Retain and grow our creator base.
Create patterns that encourage users to keep returning to Tumblr.
Improve the platform’s performance, stability, and quality.
Below is a deep dive into each of these principles.
Principle 1: Expand the ways new users can discover and sign up for Tumblr.
Tumblr has a “top of the funnel” issue in converting non-users into engaged logged-in users. We also have not invested in industry standard SEO practices to ensure a robust top of the funnel. The referral traffic that we do get from external sources is dispersed across different pages with inconsistent user experiences, which results in a missed opportunity to convert these users into regular Tumblr users. For example, users from search engines often land on pages within the blog network and blog view—where there isn’t much of a reason to sign up.
We need to experiment with logged-out tumblr.com to ensure we are capturing the highest potential conversion rate for visitors into sign-ups and log-ins. We might want to explore showing the potential future user the full breadth of content that Tumblr has to offer on our logged-out pages. We want people to be able to easily understand the potential behind Tumblr without having to navigate multiple tabs and pages to figure it out. Our current logged-out explore page does very little to help users understand “what is Tumblr.” which is a missed opportunity to get people excited about joining the site.
Actions & Next Steps
Improving Tumblr’s search engine optimization (SEO) practices to be in line with industry standards.
Experiment with logged out tumblr.com to achieve the highest conversion rate for sign-ups and log-ins, explore ways for visitors to “get” Tumblr and entice them to sign up.
Principle 2: Provide high-quality content with every app launch.
We need to ensure the highest quality user experience by presenting fresh and relevant content tailored to the user’s diverse interests during each session. If the user has a bad content experience, the fault lies with the product.
The default position should always be that the user does not know how to navigate the application. Additionally, we need to ensure that when people search for content related to their interests, it is easily accessible without any confusing limitations or unexpected roadblocks in their journey.
Being a 15-year-old brand is tough because the brand carries the baggage of a person’s preconceived impressions of Tumblr. On average, a user only sees 25 posts per session, so the first 25 posts have to convey the value of Tumblr: it is a vibrant community with lots of untapped potential. We never want to leave the user believing that Tumblr is a place that is stale and not relevant.
Actions & Next Steps
Deliver great content each time the app is opened.
Make it easier for users to understand where the vibrant communities on Tumblr are.
Improve our algorithmic ranking capabilities across all feeds.
Principle 3: Facilitate easier user participation in conversations.
Part of Tumblr’s charm lies in its capacity to showcase the evolution of conversations and the clever remarks found within reblog chains and replies. Engaging in these discussions should be enjoyable and effortless.
Unfortunately, the current way that conversations work on Tumblr across replies and reblogs is confusing for new users. The limitations around engaging with individual reblogs, replies only applying to the original post, and the inability to easily follow threaded conversations make it difficult for users to join the conversation.
Actions & Next Steps
Address the confusion within replies and reblogs.
Improve the conversational posting features around replies and reblogs.
Allow engagements on individual replies and reblogs.
Make it easier for users to follow the various conversation paths within a reblog thread.
Remove clutter in the conversation by collapsing reblog threads.
Explore the feasibility of removing duplicate reblogs within a user’s Following feed.
Principle 4: Retain and grow our creator base.
Creators are essential to the Tumblr community. However, we haven’t always had a consistent and coordinated effort around retaining, nurturing, and growing our creator base.
Being a new creator on Tumblr can be intimidating, with a high likelihood of leaving or disappointment upon sharing creations without receiving engagement or feedback. We need to ensure that we have the expected creator tools and foster the rewarding feedback loops that keep creators around and enable them to thrive.
The lack of feedback stems from the outdated decision to only show content from followed blogs on the main dashboard feed (“Following”), perpetuating a cycle where popular blogs continue to gain more visibility at the expense of helping new creators. To address this, we need to prioritize supporting and nurturing the growth of new creators on the platform.
It is also imperative that creators, like everyone on Tumblr, feel safe and in control of their experience. Whether it be an ask from the community or engagement on a post, being successful on Tumblr should never feel like a punishing experience.
Actions & Next Steps
Get creators’ new content in front of people who are interested in it.
Improve the feedback loop for creators, incentivizing them to continue posting.
Build mechanisms to protect creators from being spammed by notifications when they go viral.
Expand ways to co-create content, such as by adding the capability to embed Tumblr links in posts.
Principle 5: Create patterns that encourage users to keep returning to Tumblr.
Push notifications and emails are essential tools to increase user engagement, improve user retention, and facilitate content discovery. Our strategy of reaching out to you, the user, should be well-coordinated across product, commercial, and marketing teams.
Our messaging strategy needs to be personalized and adapt to a user’s shifting interests. Our messages should keep users in the know on the latest activity in their community, as well as keeping Tumblr top of mind as the place to go for witty takes and remixes of the latest shows and real-life events.
Most importantly, our messages should be thoughtful and should never come across as spammy.
Actions & Next Steps
Conduct an audit of our messaging strategy.
Address the issue of notifications getting too noisy; throttle, collapse or mute notifications where necessary.
Identify opportunities for personalization within our email messages.
Test what the right daily push notification limit is.
Send emails when a user has push notifications switched off.
Principle 6: Performance, stability and quality.
The stability and performance of our mobile apps have declined. There is a large backlog of production issues, with more bugs created than resolved over the last 300 days. If this continues, roughly one new unresolved production issue will be created every two days. Apps and backend systems that work well and don't crash are the foundation of a great Tumblr experience. Improving performance, stability, and quality will help us achieve sustainable operations for Tumblr.
Improve performance and stability: deliver crash-free, responsive, and fast-loading apps on Android, iOS, and web.
Improve quality: deliver the highest quality Tumblr experience to our users.
Move faster: provide APIs and services to unblock core product initiatives and launch new features coming out of Labs.
Conclusion
Our mission has always been to empower the world’s creators. We are wholly committed to ensuring Tumblr evolves in a way that supports our current users while improving areas that attract new creators, artists, and users. You deserve a digital home that works for you. You deserve the best tools and features to connect with your communities on a platform that prioritizes the easy discoverability of high-quality content. This is an invigorating time for Tumblr, and we couldn’t be more excited about our current strategy.
65K notes
·
View notes
Text
The Algorithm’s Love for Digital Conflict
Keywords: digital citizenship, online harassment, social media governance, platform accountability
Social media isn’t a town square. It’s a battleground masquerading as a forum. Platforms aren’t designed for civil discourse - they’re optimized for engagement, and nothing hooks users better than a good fight. Conflict isn’t a side effect. It’s the business model (Jenkins 2006). The more people argue, the longer they stay, the more ads they see. Everyone’s angry, but only the platform profits.
Digital spaces thrive on power struggles. It’s not just about who gets to speak - it’s about who gets heard. Social media governance claims to be neutral, but rules are dictated by corporate interests, state regulations, and decentralized moderation (Haslop, O’Rourke & Southern 2021). The result? A system where harassment is routine, outrage is currency, and moderation is inconsistent at best, weaponized at worst.
Certain groups bear the brunt of this imbalance. Plan International (2020) found that 59% of girls across 31 countries had experienced online harassment. Women, LGBTQ+ users, and racial minorities report higher levels of abuse, often with little platform intervention (Marwick & Caplan 2018). Meanwhile, bad actors manipulate reporting systems to silence dissent, reinforcing existing inequalities.
Online abuse isn’t an accident - it’s a predictable outcome of platform design. The manosphere, for instance, didn’t emerge in isolation; it was amplified by recommendation algorithms that push divisive content for engagement (Rich & Bujalka 2023). Figures like Andrew Tate didn’t rise to prominence despite their misogyny but because of it. Their rhetoric drives high engagement, making them algorithmic gold.
Moderation attempts, such as AI-driven content policing, fail to catch the nuance of harassment. Slurs might get flagged, but coordinated pile-ons, dog-whistling, and coded language slip through. Worse, enforcement is often selective. Studies show that marginalized users are disproportionately banned for calling out abuse, while harassers continue unchecked (Sundén & Paasonen 2019). The message is clear: the system isn’t broken; it’s working as intended.
Governments have tried to intervene. Australia’s Online Safety Act 2021 mandates content removal within 24 hours, but enforcement is patchy (eSafety Commissioner 2021). Platforms roll out PR-friendly initiatives - “Be Kind” campaigns, AI moderation promises - but fail to address systemic flaws. The reality? Real change rarely comes from the top down.
Instead, resistance is grassroots. Cyberfeminist movements use humor and counter-messaging to reclaim digital spaces (Dafaure 2022). Online communities document and expose abuse before it’s erased. Activists pressure advertisers, hitting platforms where it hurts - their revenue streams. Change isn’t a feature being rolled out in the next update; it’s something users have to fight for.
Social media conflict isn’t going anywhere. The real question is: who benefits? Right now, platforms profit from outrage while outsourcing the consequences to users. But awareness is growing, and so is resistance. Digital citizenship isn’t just about existing online—it’s about shaping online spaces before they shape us.
Because at the end of the day, logging off doesn’t stop the problem. It just hands the microphone to someone else.
Reference list
Dafaure, M. (2022). ‘Memes, trolls and the manosphere: Mapping the manifold expressions of antifeminism and misogyny online’, European Journal of English Studies, vol. 26, no. 2, pp. 236–254.
eSafety Commissioner. (2021). Online Safety Act 2021. Available at: https://www.esafety.gov.au.
Haslop, C., O’Rourke, F. & Southern, R. (2021). ‘#NoSnowflakes: The toleration of harassment and an emergent gender-related digital divide’, Convergence, vol. 27, no. 5, pp. 1418–1438.
Jenkins, H. (2006). Convergence Culture: Where Old and New Media Collide. New York University Press.
Marwick, A. E. & Caplan, R. (2018). ‘Drinking male tears: Language, the manosphere, and networked harassment’, Feminist Media Studies, vol. 18, no. 4, pp. 543–559.
Plan International. (2020). Free to Be Online? Girls’ and Young Women’s Experiences of Online Harassment. Available at: https://plan-international.org.
Rich, B. & Bujalka, E. (2023). ‘The draw of the ‘manosphere’: Understanding Andrew Tate’s appeal to lost men’, The Conversation, 13 February. Available at: https://theconversation.com.
Sundén, J. & Paasonen, S. (2019). ‘Inappropriate Laughter: Affective homophily and the unlikely comedy of #MeToo’, Social Media + Society. Available at: https://doi.org/10.1177/2056305119883425.
0 notes
Text
Inside the Machine: How Algorithmic Bias Fuels Echo Chambers and Filter Bubbles
Inside the Bubble: How Media Shapes What We See and Believe Echo chambers, filter bubbles, and algorithmic bias are critical frameworks in understanding how digital media influences information dissemination and consumption. These concepts address the narrowing of perspectives and reinforcement of pre-existing beliefs facilitated by modern media systems and algorithms. The term echo chamber…
0 notes
Text
Beware of Cognitive Biases in Generative AI Tools as a Reader, Researcher, or Reporter
Understanding How Human and Algorithmic Biases Shape Artificial Intelligence Outputs and What Users Can Do to Manage Them I have spent over 40 years studying human and machine cognition long before AI reached its current state of remarkable capabilities. Today, AI is leading us into uncharted territories. As a researcher focused on the ethical aspects of technology, I believe it is vital to…
#AI and Big Data Bias#AI Cognitive Biases#AI Decision-Making Risks#AI Tools for Business#Algorithmic Bias#Confirmation Bias in AI#Ethics in AI#Generative AI Bias#Human-AI Interaction#Mitigating AI Biases
0 notes
Text
Balancing AI Regulation in Education with Innovation: 6 Insights from Comparative Research
Curious about how AI is shaping the future of education? Our latest report dives into real-world insights from educators and AI experts. Discover the challenges, opportunities, and ethical considerations in AI integration.
As artificial intelligence (AI) becomes increasingly integral to educational practices, the debate over how to govern this powerful technology grows more pressing. The Organisation for Economic Co-operation and Development (OECD) recently published a working paper titled Artificial Intelligence and the Future of Work, Education, and Training, which delves into the potential impact of AI on equity…
#AI governance#AI in classrooms#AI in education#AI policy#AI regulation#algorithmic bias#data privacy#Ethical AI#Graeme Smith#Innovation in Education#OECD AI report#thisisgraeme
0 notes
Text
AI Bias: Why Algorithmic Bias can hurt your business? - Bionic
This Blog was Originally Published at:
AI Bias: Why Algorithmic Bias can hurt your business? — Bionic
A decade ago, two individuals, Brisha Borden and Vernon Prater, found themselves entangled with the law. While Borden, an 18-year-old Black woman, was arrested for riding an unlocked bike, Prater, a 41-year-old white man with a criminal history, was caught shoplifting $86,000 worth of tools.
Yet, when assessed by a supposedly objective AI algorithm in the federal jail, Borden was deemed high-risk, while Prater was labeled low-risk. Two years later, Borden remained crime-free, while Prater was back behind bars.
This stark disparity exposed a chilling truth: the algorithm’s risk assessments were racially biased, favoring white individuals over Black individuals, despite claims of objectivity. This is just one of the many AI bias examples, the tendency of AI systems to produce systematically unfair outcomes due to inherent flaws in their design or the data they are trained on.
Things haven’t changed much since then. Even when explicit features like race or gender are omitted, AI algorithms can still perpetuate discrimination by drawing correlations from data points like schools or neighborhoods. This often comes with historical human biases embedded in the data they are trained on.
AI is good at describing the world as it is today with all of its biases, but it does not know how the world should be.” — Joanne Chen
To fully realize the potential of AI in the interest of business while minimizing its potential for negative effects, it is crucial to recognize its potential drawbacks, take measures to address its negative effects and understand its roots.
In this article, we will take a closer look at the bear traps of AI and algorithmic bias, understand its types, and discuss the negative impacts it can have on your company. We will also teach you how to develop fair AI systems that contribute to the general welfare of society.
Indeed, the future of AI should not be defined by the perpetuation of algorithmic bias but by striving for the greater good and fairness for everyone.
What is AI Bias?
AI biases occur when artificial intelligence systems produce results that are systematically prejudiced due to flawed data, algorithm design, or even unintentional human influence.
For instance, COMPAS is an AI technology employed by US courts to assess the risk of a defendant committing further crimes. Like any other risk-assessment tool, COMPAS was used and was condemned for being racially prejudiced, as it more often labeled black defendants as high risk than white ones with similar criminal records.

Understanding the Roots of Algorithmic Bias
Machine learning bias is often inherent and not brought in as a flaw; it simply mirrors our societal prejudices that are fed into it.
These biases may not always be bad for the human mind because they can help someone make quick decisions in a certain situation. On the other hand, when such biases are included or incorporated into AI systems, the results may be disastrous.
Think of AI as a sponge that absorbs the data it is trained on; if the data contains prejudice that exists within society, the AI will gradually incorporate those prejudices. The incomplete training data also makes the AI come up with AI hallucinations, which basically are AI systems generating weird or inaccurate results due to incomplete training data.
The data that machine learning bias flourishes in is either historical data, which captures past injustices, or current data with skewed data distribution that fails to include marginalized groups. This can happen if one uses the Grounding AI approach based on biased training data or the design of algorithms themselves. Algorithmic bias can arise from the choices made by developers, the assumptions they make, and the data they choose to use.
The issue, therefore, is to identify and address such biased sources before they create a problem. It is about making sure that the training data, for AI models, is as diverse and inclusive as the real world, and does not contain prejudice.
The Ripple Effects of AI Bias in Business
Algorithmic bias can lead to discriminatory outcomes in hiring, lending, and other critical business processes
AI bias isn’t confined to theoretical discussions or academic debates. It has a real and measurable impact on the bottom line of businesses, leaving a trail of financial losses, legal battles, and tarnished reputations.
Reputational Damage: Consider the cautionary tale of Microsoft’s AI chatbot, Tay. Within hours of its release in 2016, Tay, trained on Twitter conversations, learned to spew racist and sexist remarks, forcing Microsoft to quickly shut it down. The incident not only showcased the dangers of unchecked machine learning bias but also dealt a significant blow to Microsoft’s reputation, raising concerns about its commitment to ethical AI development. (Know more)
Financial Losses: The consequences of this algorithmic bias are not only social but also have financial implications that are just as severe. Another high-profile scandal involving Goldman Sachs surfaced in 2019 when it was revealed that its Apple Card was programmed to provide significantly lower credit limits to women than their male counterparts with similar credit scores and incomes. This led to outrage, demands for an inquiry, and possible legal proceedings showcasing the fact that bias in AI software has severe financial repercussions. (Know More)
Legal Troubles: Legal troubles are another equally grave problem. Facebook was accused of discrimination in housing through its ad-targeting platform, which was claimed to demographically exclude individuals of color, women, and persons with disabilities, among others. This case shows how companies expose themselves to legal risks when their AI systems reproduce bias. (Know More)
Eroded Customer Trust: Algorithmic bias also has significant social impacts: it may lead to loss of customer confidence, which is a crucial component in any company. A Forbes Advisor survey shows that 76% of consumers are concerned with misinformation from artificial intelligence (AI) tools such as Google Bard, ChatGPT, and Bing Chat. This lack of trust translates to a loss in sales, customers and clients switching to other better firms, and erosion of brand image. (Know More)
A Multi-Pronged Approach to Tackle AI Bias
Mitigating AI bias requires a holistic approach, addressing both technical and organizational factors:
Data Diversity: Make sure training data is as diverse as possible to meet real-world applications. This includes using sources to gather information and making sure everyone who needs to be represented has been included.
Algorithmic Transparency: Introduce AI systems that are understandable so that users can see how decisions are being made. This helps to ensure that biases are detected and eradicated where necessary.
Bias Testing and Auditing: Biases should be detected in AI systems periodically through some automated methods, and they should be checked by reviewers as well. It is advisable to engage several stakeholders in this process as this will provide the needed diversity of opinion.
Ethical Frameworks: Implement best practices and standards that shield your organization from core ethical risks associated with AI. Enhance the culture of accountability and responsibility.
Human-in-the-Loop: Always have human supervision during the development of an AI system, from its design to deployment. AI can perform tasks independently, but when it comes to correcting algorithmic bias, it is the judgment of a human that is needed. This is called having a human in the loop of AI training.
Conclusion
Creating ethical AI isn’t a one-and-done deal; it’s a constant balancing act that requires our unwavering attention. We must first acknowledge the uncomfortable truth: bias is deeply ingrained in our society and can easily infiltrate the very AI technology we create.
This means we need to build diverse teams, bringing together people with different backgrounds, experiences, and perspectives.
Shining a light on AI’s decision-making process is equally important. We need to understand why AI makes the choices it does. Transparency builds trust, ensures accountability, and makes it easier to spot and correct potential biases.
But technology alone can’t solve this problem. We need strong ethical frameworks, a shared sense of responsibility, and clear rules for AI development. After all, the people behind the technology, the environment in which it’s created, and the values it embodies will ultimately determine whether AI helps or hinders humanity.
Don’t let bias hold back the potential of AI. Embrace the power of Bionic AI to unlock a future where innovation and ethics go hand in hand. Book a demo now!
1 note
·
View note
Text
Unlocking Insights: How Machine Learning Is Transforming Big Data
Introduction
Big data and machine learning are two of the most transformative technologies of our time. At TechtoIO, we delve into how machine learning is revolutionizing the way we analyze and utilize big data. From improving business processes to driving innovation, the combination of these technologies is unlocking new insights and opportunities. Read to continue
#Tech Trends#TagsAI and big data#algorithmic bias#big data#big data analysis#customer insights#data processing#data quality#data security#ethical considerations in AI#fraud detection#healthcare innovations#IoT and machine learning#machine learning#machine learning applications#machine learning trends#predictive analytics#Technology#Science#business tech#Adobe cloud#Trends#Nvidia Drive#Analysis#Tech news#Science updates#Digital advancements#Tech trends#Science breakthroughs#Data analysis
1 note
·
View note